You can not select more than 25 topics
Topics must start with a letter or number, can include dashes ('-') and can be up to 35 characters long.
127 lines
6.1 KiB
127 lines
6.1 KiB
--- |
|
comments: true |
|
description: Instance Segmentation with Object Tracking using Ultralytics YOLOv8 |
|
keywords: Ultralytics, YOLOv8, Instance Segmentation, Object Detection, Object Tracking, Segbbox, Computer Vision, Notebook, IPython Kernel, CLI, Python SDK |
|
--- |
|
|
|
# Instance Segmentation and Tracking using Ultralytics YOLOv8 🚀 |
|
|
|
## What is Instance Segmentation? |
|
|
|
[Ultralytics YOLOv8](https://github.com/ultralytics/ultralytics/) instance segmentation involves identifying and outlining individual objects in an image, providing a detailed understanding of spatial distribution. Unlike semantic segmentation, it uniquely labels and precisely delineates each object, crucial for tasks like object detection and medical imaging. |
|
|
|
There are two types of instance segmentation tracking available in the Ultralytics package: |
|
|
|
- **Instance Segmentation with Class Objects:** Each class object is assigned a unique color for clear visual separation. |
|
|
|
- **Instance Segmentation with Object Tracks:** Every track is represented by a distinct color, facilitating easy identification and tracking. |
|
|
|
## Samples |
|
|
|
| Instance Segmentation | Instance Segmentation + Object Tracking | |
|
|:---------------------------------------------------------------------------------------------------------------------------------------:|:------------------------------------------------------------------------------------------------------------------------------------------------------------:| |
|
| 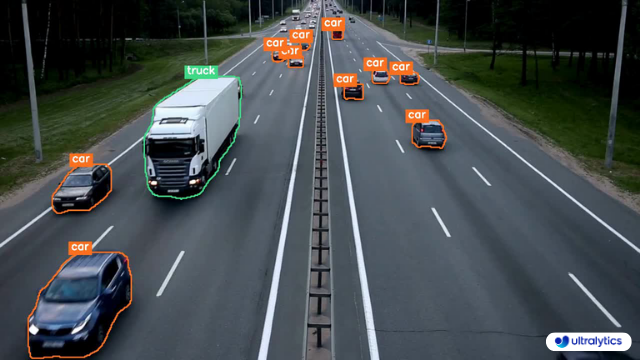 | 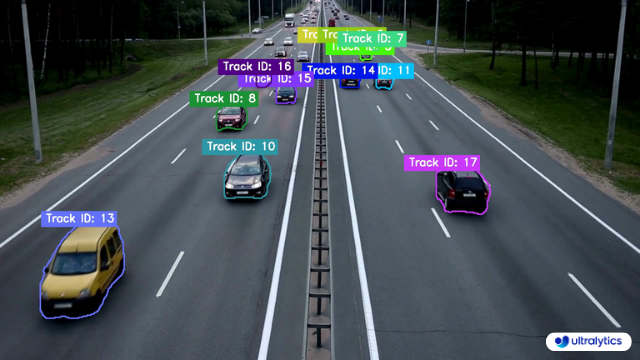 | |
|
| Ultralytics Instance Segmentation 😍 | Ultralytics Instance Segmentation with Object Tracking 🔥 | |
|
|
|
!!! Example "Instance Segmentation and Tracking" |
|
|
|
=== "Instance Segmentation" |
|
```python |
|
import cv2 |
|
from ultralytics import YOLO |
|
from ultralytics.utils.plotting import Annotator, colors |
|
|
|
model = YOLO("yolov8n-seg.pt") # segmentation model |
|
names = model.model.names |
|
cap = cv2.VideoCapture("path/to/video/file.mp4") |
|
w, h, fps = (int(cap.get(x)) for x in (cv2.CAP_PROP_FRAME_WIDTH, cv2.CAP_PROP_FRAME_HEIGHT, cv2.CAP_PROP_FPS)) |
|
|
|
out = cv2.VideoWriter('instance-segmentation.avi', cv2.VideoWriter_fourcc(*'MJPG'), fps, (w, h)) |
|
|
|
while True: |
|
ret, im0 = cap.read() |
|
if not ret: |
|
print("Video frame is empty or video processing has been successfully completed.") |
|
break |
|
|
|
results = model.predict(im0) |
|
annotator = Annotator(im0, line_width=2) |
|
|
|
if results[0].masks is not None: |
|
clss = results[0].boxes.cls.cpu().tolist() |
|
masks = results[0].masks.xy |
|
for mask, cls in zip(masks, clss): |
|
annotator.seg_bbox(mask=mask, |
|
mask_color=colors(int(cls), True), |
|
det_label=names[int(cls)]) |
|
|
|
out.write(im0) |
|
cv2.imshow("instance-segmentation", im0) |
|
|
|
if cv2.waitKey(1) & 0xFF == ord('q'): |
|
break |
|
|
|
out.release() |
|
cap.release() |
|
cv2.destroyAllWindows() |
|
|
|
``` |
|
|
|
=== "Instance Segmentation with Object Tracking" |
|
```python |
|
import cv2 |
|
from ultralytics import YOLO |
|
from ultralytics.utils.plotting import Annotator, colors |
|
|
|
from collections import defaultdict |
|
|
|
track_history = defaultdict(lambda: []) |
|
|
|
model = YOLO("yolov8n-seg.pt") # segmentation model |
|
cap = cv2.VideoCapture("path/to/video/file.mp4") |
|
w, h, fps = (int(cap.get(x)) for x in (cv2.CAP_PROP_FRAME_WIDTH, cv2.CAP_PROP_FRAME_HEIGHT, cv2.CAP_PROP_FPS)) |
|
|
|
out = cv2.VideoWriter('instance-segmentation-object-tracking.avi', cv2.VideoWriter_fourcc(*'MJPG'), fps, (w, h)) |
|
|
|
while True: |
|
ret, im0 = cap.read() |
|
if not ret: |
|
print("Video frame is empty or video processing has been successfully completed.") |
|
break |
|
|
|
annotator = Annotator(im0, line_width=2) |
|
|
|
results = model.track(im0, persist=True) |
|
|
|
if results[0].boxes.id is not None and results[0].masks is not None: |
|
masks = results[0].masks.xy |
|
track_ids = results[0].boxes.id.int().cpu().tolist() |
|
|
|
for mask, track_id in zip(masks, track_ids): |
|
annotator.seg_bbox(mask=mask, |
|
mask_color=colors(track_id, True), |
|
track_label=str(track_id)) |
|
|
|
out.write(im0) |
|
cv2.imshow("instance-segmentation-object-tracking", im0) |
|
|
|
if cv2.waitKey(1) & 0xFF == ord('q'): |
|
break |
|
|
|
out.release() |
|
cap.release() |
|
cv2.destroyAllWindows() |
|
``` |
|
|
|
### `seg_bbox` Arguments |
|
|
|
| Name | Type | Default | Description | |
|
|---------------|---------|-----------------|----------------------------------------| |
|
| `mask` | `array` | `None` | Segmentation mask coordinates | |
|
| `mask_color` | `tuple` | `(255, 0, 255)` | Mask color for every segmented box | |
|
| `det_label` | `str` | `None` | Label for segmented object | |
|
| `track_label` | `str` | `None` | Label for segmented and tracked object | |
|
|
|
## Note |
|
|
|
For any inquiries, feel free to post your questions in the [Ultralytics Issue Section](https://github.com/ultralytics/ultralytics/issues/new/choose) or the discussion section mentioned below.
|
|
|